Using auto scaling for Amazon Glue
Auto Scaling is available for your Amazon Glue ETL, interactive sessions, and streaming jobs with Amazon Glue version 3.0 or later.
With Auto Scaling enabled, you will get the following benefits:
-
Amazon Glue automatically adds and removes workers from the cluster depending on the parallelism at each stage or microbatch of the job run.
-
It removes the need for you to experiment and decide on the number of workers to assign for your Amazon Glue ETL jobs.
-
If you choose the maximum number of workers, Amazon Glue will choose the right size resources for the workload.
-
You can see how the size of the cluster changes during the job run by looking at CloudWatch metrics on the job run details page in Amazon Glue Studio.
Auto Scaling for Amazon Glue ETL and streaming jobs enables on-demand scaling up and scaling down of the computing resources of your Amazon Glue jobs. On-demand scale-up helps you to only allocate the required computing resources initially on job run startup, and also to provision the required resources as per demand during the job.
Auto Scaling also supports dynamic scale-down of the Amazon Glue job resources over the course of a job. Over a job run, when more executors are requested by your Spark application, more workers will be added to the cluster. When the executor has been idle without active computation tasks, the executor and the corresponding worker will be removed.
Common scenarios where Auto Scaling helps with cost and utilization for your Spark applications include a Spark driver listing a large number of files in Amazon S3 or performing a load while executors are inactive, Spark stages running with only a few executors due to overprovisioning, and data skews or uneven computation demand across Spark stages.
Requirements
Auto Scaling is only available for Amazon Glue version 3.0 or later. To use Auto Scaling, you can follow the migration guide to migrate your existing jobs to Amazon Glue version 3.0 or later or create new jobs with Amazon Glue version 3.0 or later.
Auto Scaling is available for Amazon Glue jobs with the G.1X
,
G.2X
, G.4X
, G.8X
, or G.025X
(only for Streaming jobs) worker types. Standard DPUs are not
supported.
Enabling Auto Scaling in Amazon Glue Studio
On the Job details tab in Amazon Glue Studio, choose the
type as Spark or Spark Streaming, and
Glue version as Glue 3.0
or
Glue 4.0
. Then a check box will show up below
Worker type.
-
Select the Automatically scale the number of workers option.
-
Set the Maximum number of workers to define the maximum number of workers that can be vended to the job run.
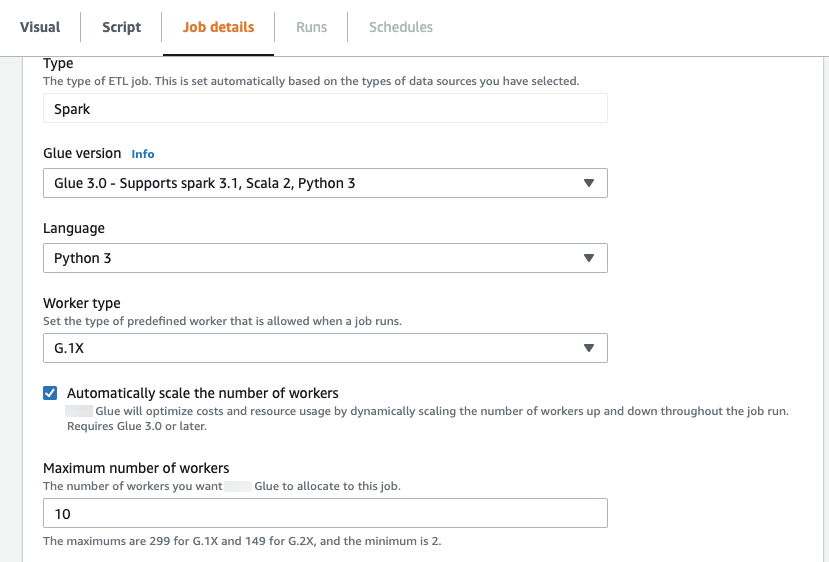
Enabling Auto Scaling with the Amazon CLI or SDK
To enable Auto Scaling from the Amazon CLI for your job run, run
start-job-run
with the following configuration:
{ "JobName": "<your job name>", "Arguments": { "--enable-auto-scaling": "true" }, "WorkerType": "G.2X", // G.1X and G.2X are allowed for Auto Scaling Jobs "NumberOfWorkers": 20, // represents Maximum number of workers ...other job run configurations... }
Once at ETL job run is finished, you can also call get-job-run
to check
the actual resource usage of the job run in DPU-seconds. Note: the new field
DPUSeconds will only show up for your batch jobs on Amazon Glue 3.0
or later enabled with Auto Scaling. This field is not supported for streaming
jobs.
$ aws glue get-job-run --job-name your-job-name --run-id jr_xx --endpoint https://glue.us-east-1.amazonaws.com --region us-east-1 { "JobRun": { ... "GlueVersion": "3.0", "DPUSeconds": 386.0 } }
You can also configure job runs with Auto Scaling using the Amazon Glue
SDK
Enabling Auto Scaling with Interactive sessions
To enable Auto Scaling when building Amazon Glue jobs with interactive sessions, see Configuring Amazon Glue interactive sessions.
Monitoring Auto Scaling with Amazon CloudWatch metrics
The CloudWatch executor metrics are available for your Amazon Glue 3.0 or later jobs if you enable Auto Scaling. The metrics can be used to monitor the demand and optimized usage of executors in their Spark applications enabled with Auto Scaling. For more information, see Monitoring Amazon Glue using Amazon CloudWatch metrics.
Note
The following CloudWatch executor metrics are not available for interactive sessions.
-
glue.driver.ExecutorAllocationManager.executors.numberAllExecutors
-
glue.driver.ExecutorAllocationManager.executors.numberMaxNeededExecutors
For more details on these metrics, see Monitoring for DPU capacity planning.
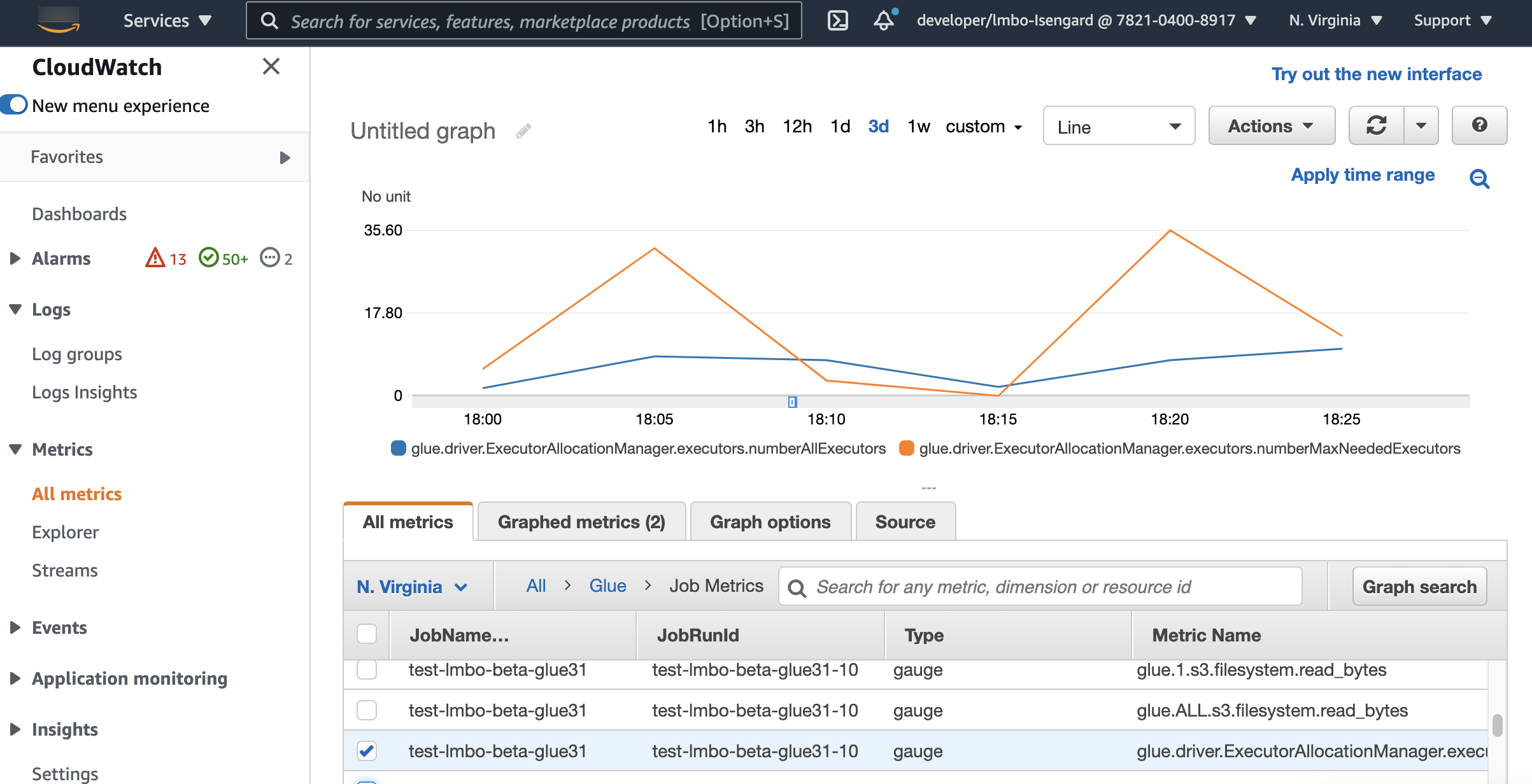
If you are using interactive sessions, you can monitor the number of executors by enabling continuous Amazon CloudWatch logs and
searching “executor” in the logs, or by using the Spark UI. To do this, use the %%configure
magic to enable
continuous Amazon CloudWatch Logs and enable auto scaling.
%%configure { “--enable-continuous-cloudwatch-log”: “true”, “--enable-auto-scaling”: “true” }
In the Amazon CloudWatch log events, search "executor"in the logs:
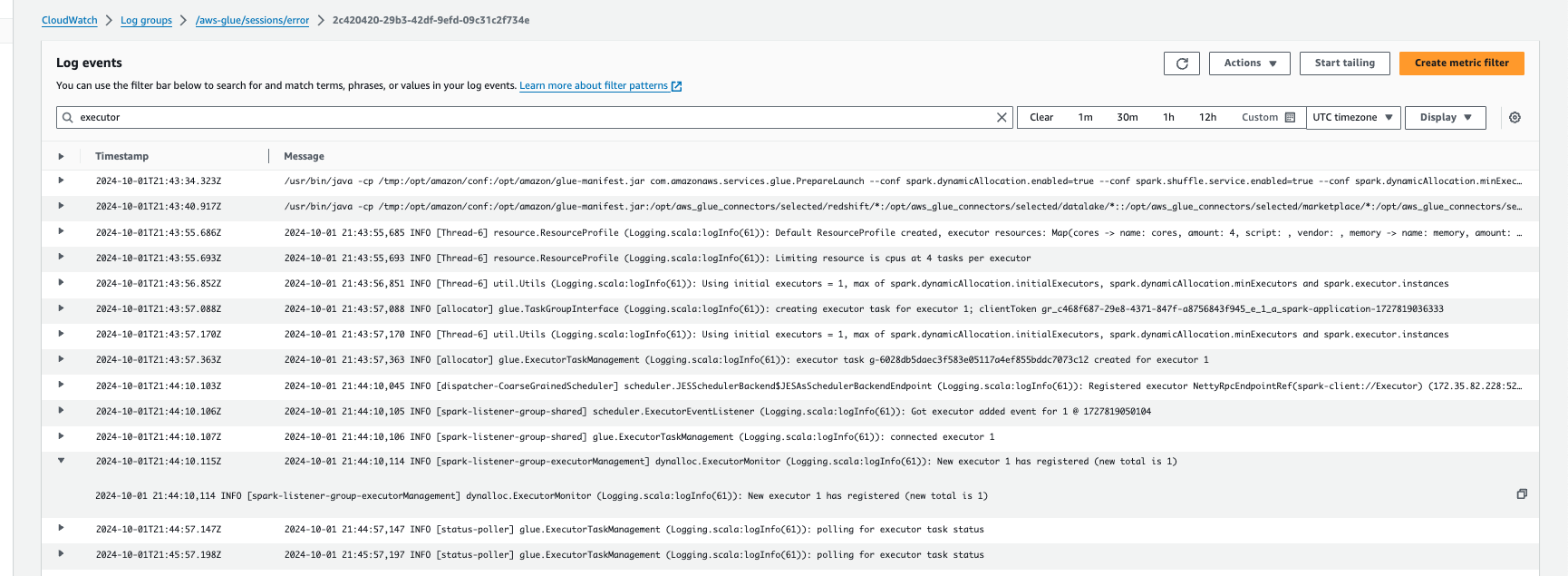
Monitoring Auto Scaling with Spark UI
With Auto Scaling enabled, you can also monitor executors being added and removed with dynamic scale-up and scale-down based on the demand in your Amazon Glue jobs using the Amazon Glue Spark UI. For more information, see Enabling the Apache Spark web UI for Amazon Glue jobs.
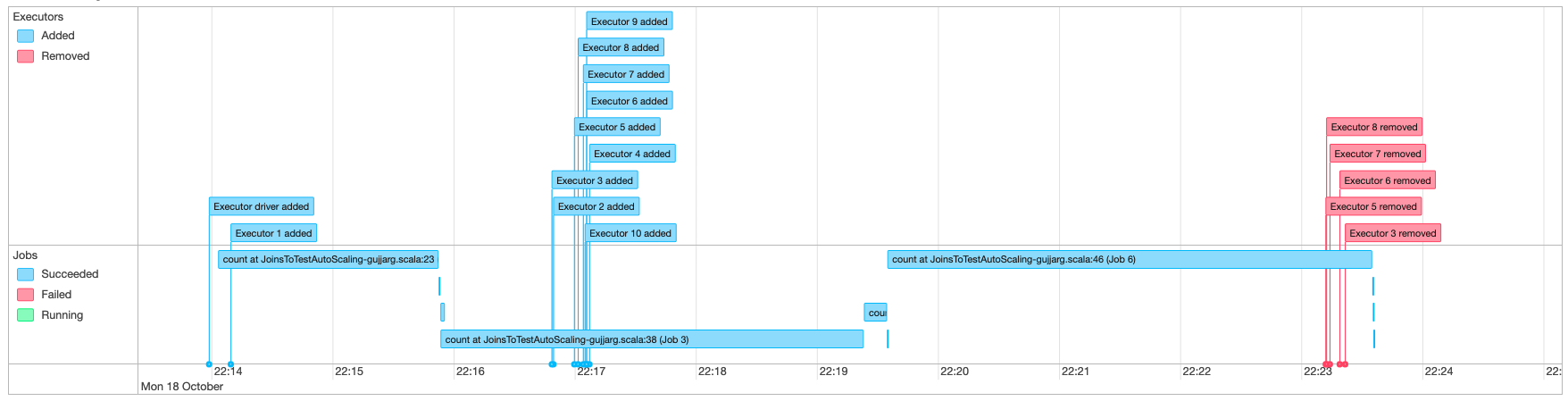
Monitoring Auto Scaling job run DPU usage
You may use the Amazon Glue Studio Job
run view
-
Choose Monitoring from the Amazon Glue Studio navigation pane. The Monitoring page appears.
-
Scroll down to the Job runs chart.
-
Navigate to the job run you are interested and scroll to the DPU hours column to check the usage for the specific job run.
Limitations
Amazon Glue streaming Auto Scaling currently doesn't support a streaming
DataFrame join with a static DataFrame created outside of ForEachBatch
. A
static DataFrame created inside the ForEachBatch
will work as
expected.